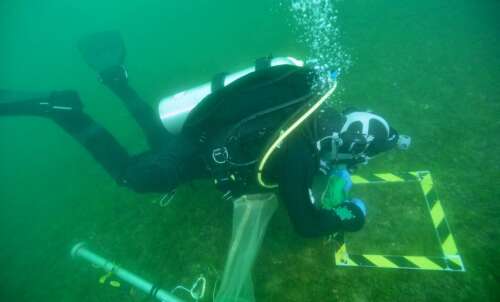
Better monitoring of invasive mussels in the Great Lakes is the long-term goal of a novel University of Guelph project using computer vision and artificial intelligence (AI).
In what they believe is the first such application of machine learning, the research team has shown that AI can scan images, pick out mussels from the pictures and automatically map where and how many of the ecologically damaging mollusks cover the bottom of freshwater lakes.
Tracking the whereabouts and abundance of zebra mussels and quagga mussels is vital for preventing biofouling of drinking water intakes and other infrastructure and for addressing other indirect problems such as nuisance algal growth that come with the bottom-living creatures.
The researchers say their work holds promise for improving AI and computer vision systems for efficient, consistent mapping and could help relieve human scuba divers of part of the laborious task of estimating mussel abundance by hand.
“We’re one of the first to show that this is feasible,” said Angus Galloway, a PhD candidate in the School of Engineering within U of G’s College of Engineering and Physical Sciences. “Now we want to optimize the accuracy of the method.”
He is first author of a paper that recently appeared in the journal Limnology and Oceanography Methods. Co-authors were U of G engineers, including Dr. Graham Taylor and Dr. Medhat Moussa, and scientists with Environment and Climate Change Canada (ECCC).
Machine learning uses AI to recognize, estimate mussel numbers
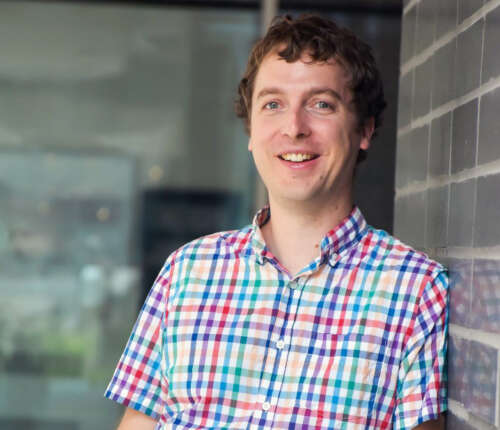
Taylor’s lab studies machine learning, which uses AI models to tackle huge amounts of data to improve the performance of predictive algorithms in various applications. Taylor is academic co-director of U of G’s Centre for Advancing Responsible and Ethical AI and interim research director at the Vector Institute for Artificial Intelligence based in Toronto.
For this study, the team trained a computer model to recognize mussels from 1,600 photos taken in the northeastern end of Lake Erie and western Lake Ontario. Snapped between 2016 and 2018, the images were taken by divers using window-sized metal sampling frames laid on the lake bottom to gauge mussel abundance, coverage and biomass.
Counting mussels accurately and efficiently can be a challenging task for people or computers. Underwater conditions may be less than ideal, with mussels half-buried in mud or with suspended sediments or vegetation obscuring the view.
Taylor said, “This task of counting and estimating mussel biomass is where I think AI will have an advantage due to the difficult underwater conditions under which these images are taken.”
Working hands-on, divers proved better at gauging mussel biomass (93 per cent accurate) and abundance (91 per cent) than the machine learning system (80 and 71 per cent, respectively). “There’s room for improvement in the model,” says Galloway.
The system fared better when compared against trial assessments of almost 200 images pored over by Galloway and ECCC technicians. Estimates of mussel coverage by AI were close to those of the human team.
“The model predictions are well correlated with human intuition.”
Toward better mapping, monitoring, control of invasive mussels
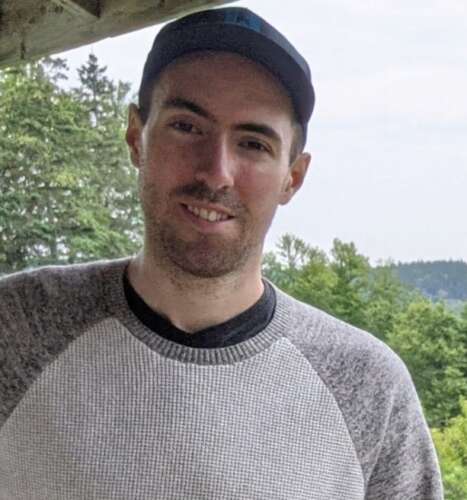
Galloway said such a system might be used to complement divers’ assessments of the mussel problem. Diving can be expensive, he said. He envisions cameras towed by a boat or affixed to a remotely operated vehicle cruising along the lake bottom to capture images.
“The advantage of this method is that it’s real time,” he said, referring to the system’s ability to instantaneously share image information data and speed up image analysis. “It’s analyzing data as quickly as it’s collecting it in a non-contact way.”
Ideally, he said, the information would enable authorities to better map and monitor changes in mussel abundance and ultimately control them in the Great Lakes. “They completely carpet the bottom in many locations. The maps we have are a sparse representation.”
Through the Vector Institute, ECCC scientists had contacted U of G about prospects for analyzing their collected lake bottom images. Earlier, Galloway had used similar technology to detect and control invasive invertebrates called tunicates on blue mussels farmed for food in Prince Edward Island.
He said the U of G model might be adapted for monitoring other invasive aquatic species and perhaps for use in drones for land surveillance.
Contact:
Dr. Graham Taylor
gwtaylor@uoguelph.ca