From more precise medical imaging to improved drug design, numerous benefits may ultimately arise from an innovation by University of Guelph researchers that enables much faster machine learning than ever.
The new method for training so-called neural networks in seconds rather than the typical hours or days is also intended to “democratize” machine learning by making the technology accessible to smaller players in this growing field, said Dr. Graham Taylor, an engineering professor and machine learning expert in the College of Engineering and Physical Sciences.
The innovation is described in a peer-reviewed paper recently posted online. The study was presented at last month’s virtual NeurIPS 2021, the Conference on Neural Information Processing Systems.
Taylor worked on the study with first author and PhD student Boris Knyazev, along with experts at the Vector Institute for Artificial Intelligence at the University of Toronto and Facebook AI Research (now renamed Meta AI).
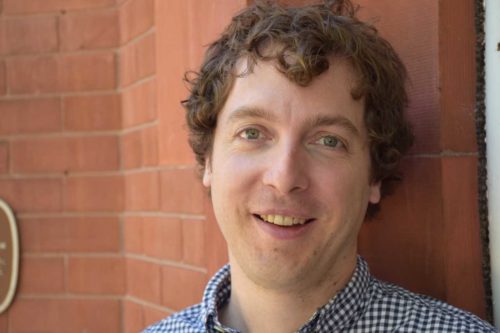
Like an artificial brain, neural networks enable computers to learn and make predictions from piles of data. So-called “deep learning” underlies artificial intelligence systems used in varied applications from digital assistants to self-driving cars.
Training such a system usually takes hours or days as the computer brain learns, say, to distinguish objects by analyzing huge numbers of images. “That takes a long time to do,” said Taylor, director of the Centre for Advancing Responsible and Ethical Artificial Intelligence at U of G. “It’s also expensive. There are a lot of barriers to working with these larger neural networks.”
Referring to the new training method, he said, “It’s really surprising to us that it works so well. We can achieve in a second what would normally take hours or days to do.”
Taylor is especially interested in potential health uses, from machines able to analyze medical images to AI systems that can crunch through numerous complex molecules to find drug design candidates.
The project was led by Knyazev, who completed a summer internship with Facebook (now Meta) in 2020 as part of his research.
‘To democratize machine learning is my motivation’
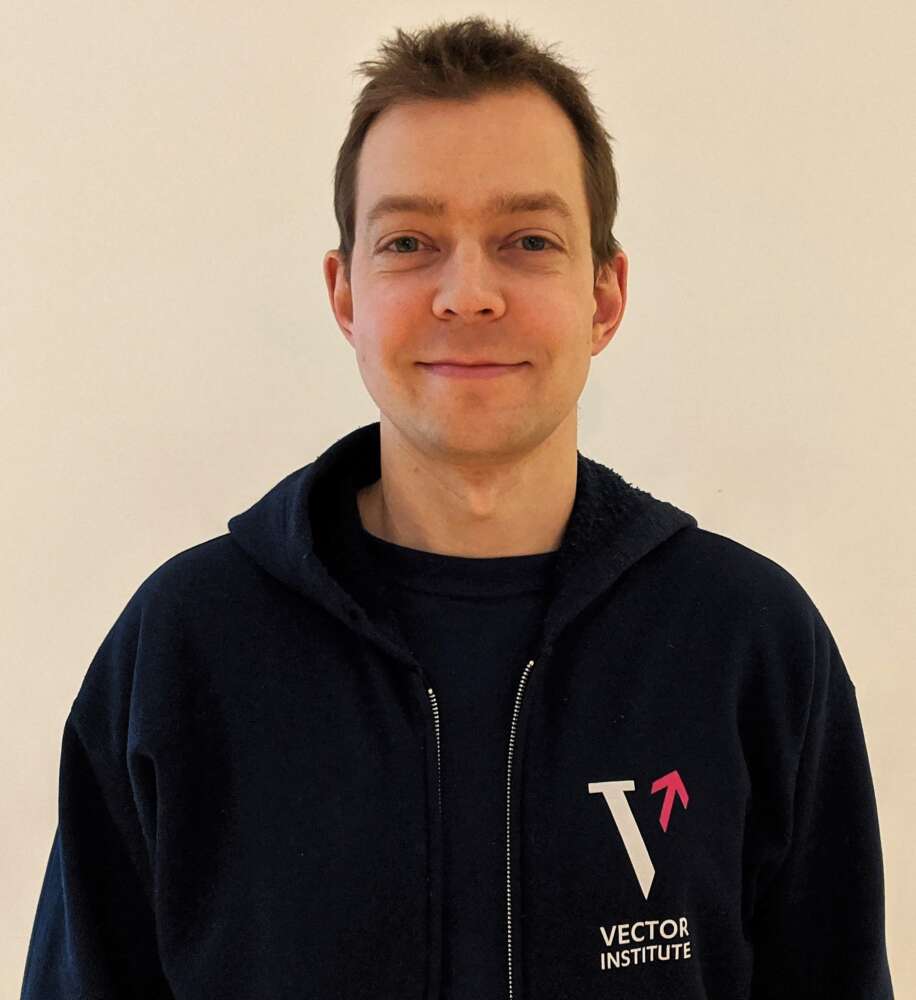
In developing a faster-working machine brain, he aimed to share his innovation with other researchers and developers of all sizes beyond big players such as Google or Facebook.
“I think it’s a little unfair when some people can use many resources to train big models while others cannot,” said Knyazev, who will continue his doctorate while working as a full-time researcher with Samsung’s AI lab in Montreal. “To democratize machine learning is my motivation. Also: scientific curiosity. People think it’s a crazy, unsolvable task.”
The new paper describes a method for machines that effectively uses two levels of neural networks – what Taylor calls a teacher network and numerous student networks.
He likens the system to a teacher who could bypass a preschooler’s early years of experiencing the world by instantaneously injecting a four-year-old’s knowledge into a newborn’s brain.
Knyazev said the team needs to do further research to refine the model for real-world use. However, online release of the results has sparked interest from various developers – including numerous likes on Twitter — who may be interested in using their work for eventual applications, he said.
“I think this is a very important step toward future directions where we will be able to train similar models that can be applied to many different tasks.”
Contact:
Dr. Graham Taylor
gwtaylor@uoguelph.ca