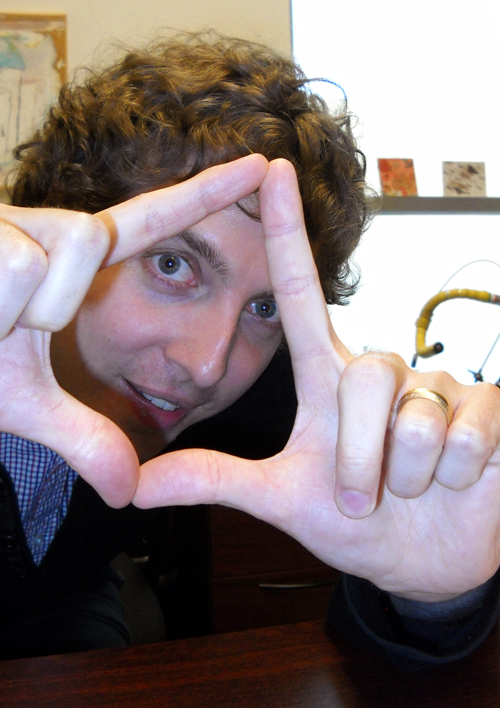
How often do you get a band to collect your research data for you? That’s more or less what Graham Taylor and other researchers at New York University (NYU) did two years ago.
Today Taylor is a professor in U of G’s School of Engineering. In 2011, he was still a post-doc at NYU’s Courant School of Mathematical Sciences, looking for data to study machine learning. Not just any data, but images of people all posed the same way – lots of people and lots of images to help train computers to “see” better.
“We thought: ‘How to collect a data set with people posing in the same way?’ We were interested in imitation. Just as we were thinking about imitation, this band was doing that.”
C-Mon & Kypski, a Dutch progressive electronic group, was making a new crowd-sourcing video for a particular song and had asked fans for help. Their request: look at a frame from the video, copy the pose with a webcam and send us the image.
By the time Taylor and his collaborators tapped in – with the band’s permission – the group had collected more than 25,000 images. “We took all the images from the video to train the system,” he says.
Now at Guelph, he’s working not with musicians but with plant and environmental scientists. But the goals are the same: to help develop smarter computers whose brains work more like our own.
It’s called “deep learning,” or using algorithms based on representations to help machines learn. You recognize someone’s face without thinking about it. Or you can name a song after hearing only a few bars. “We have this amazing ability to do that recognition,” says Taylor. “Machine learning is about getting machines to do what people are good at.”
For a computer, that kind of feat requires a lot of “thought.” You might try to train the machine to recognize faces by feeding it lots of representations of images, some more useful than others.
Taylor and others are looking for the optimum ones in order to help computers learn. Traditionally, that learning has involved digital pixels and ones and zeroes. What other representations – colours or shapes – might be used to train computers to recognize your face?
“We don’t think in numbers,” says the U of G engineer. Indeed, he and other scientists believe training computers to think more like biological machines will not only solve the problem of artificial intelligence but will also give us insights into how the human brain works.
He says these ideas are already yielding machines that can do “amazing things.” Look at how Google Street View can – virtually – land you anywhere in the world. Or how recommender systems such as Netflix and Pandora Radio can offer choices to Taylor based on his tastes for, say, the Arrested Development TV series, the Danish film The Celebration or the Elephant 6 music collective. “When you search on Google, there’s machine understanding of language involved.”
Another sign of changes afoot is that computer scientists, engineers and neuroscientists are entering one another’s orbit, he says, including neuroscientists being hired at Google.
Taylor spent two years in that NYU post-doc working with Yann LeCun, Rob Fergus and Chris Bregler. He had completed a PhD at the University of Toronto with Geoff Hinton, following an undergrad and master’s degree at the University of Waterloo. During his master’s degree, he studied at INSA Lyon in France.
Interested in applying deep learning to different fields, he came to Guelph in 2012. He sees opportunities here to develop and use smarter machines in biology, agriculture, environment and food.
He has already worked with Prof. Rebecca Hallett, School of Environmental Sciences, on a prototype for “smart” insect traps. Along with a private company, they’re testing a camera inside a field trap that will identify insect pests without needing to send samples to experts. Wielding a sample box in his Thornbrough Building office, he says, “It’s a difficult recognition problem.”
Along with plant agriculture professor Ralph Martin, Taylor hopes to make smarter unmanned aerial vehicles (UAV) for surveillance of farm fields, an area of growing interest for crop specialists. “I would develop algorithms to automatically understand image data from the UAV,” he says.
That would allow researchers and farmers to remotely gauge anything from crop growth to pest problems to soil moisture. This project also involves geography professor Aaron Berg, who already works with North American researchers on soil moisture data collected by satellite.
Says Taylor: “Deep learning is one of those fields that can impact different disciplines. Everyone is generating lots of data and everybody wants to do more with data.”
He grew up in London, Ont., where he developed an early fascination for computers. “I got my first Commodore when I was six and started programming. All through school I was absorbed with computers.” He studied systems design at Waterloo, where he teamed up with other students to devise award-winning software for computer gaming.
“A lot of people are interested in artificial intelligence and machine learning for teaching,” he says. That might include his wife, Andria Natale, a high school teacher. Their first child was born in June.
It might also include Taylor’s sister, Meredith, who did child studies at Guelph and now works with children as a speech language pathologist in London. For kids lacking speech, he says, “I think AI holds promise for people to communicate with the world.”